As a data science enthusiast, I’m always intrigued by the intersection of sports and analytics. In this article, I delve into the exciting realm of predicting Champions League game outcomes using data-driven insights. By harnessing the power of statistical models and machine learning algorithms, we can uncover trends and patterns that offer a glimpse into how these high-stakes matches might unfold.
I’ll explore the fascinating world of sports analytics and demonstrate how data science can be a game-changer in forecasting the results of Champions League fixtures. From analyzing player performance metrics to evaluating team strategies, the possibilities are endless when it comes to leveraging data to anticipate the outcomes of these thrilling football encounters. Join me on this insightful journey as we unlock the potential of data science in predicting the fate of Champions League games.
The Role of Data Science in Predicting Champions League Outcomes
Understanding Predictive Modeling
In the realm of predicting Champions League outcomes, data science plays a pivotal role through the lens of predictive modeling. By leveraging historical data, statistical analysis, and machine learning algorithms, https://india1xbet.in/en I extract valuable insights to forecast match results accurately. Through advanced statistical techniques, I delve into team performance, player statistics, and various gameplay aspects to create models that predict the potential outcomes of Champions League games.
Key Metrics Analyzed in Football
When delving into the prediction of Champions League games, certain key metrics stand out as crucial factors in the analysis. I focus on metrics such as possession percentage, successful passes, shots on target, goal conversion rates, and defensive solidity.
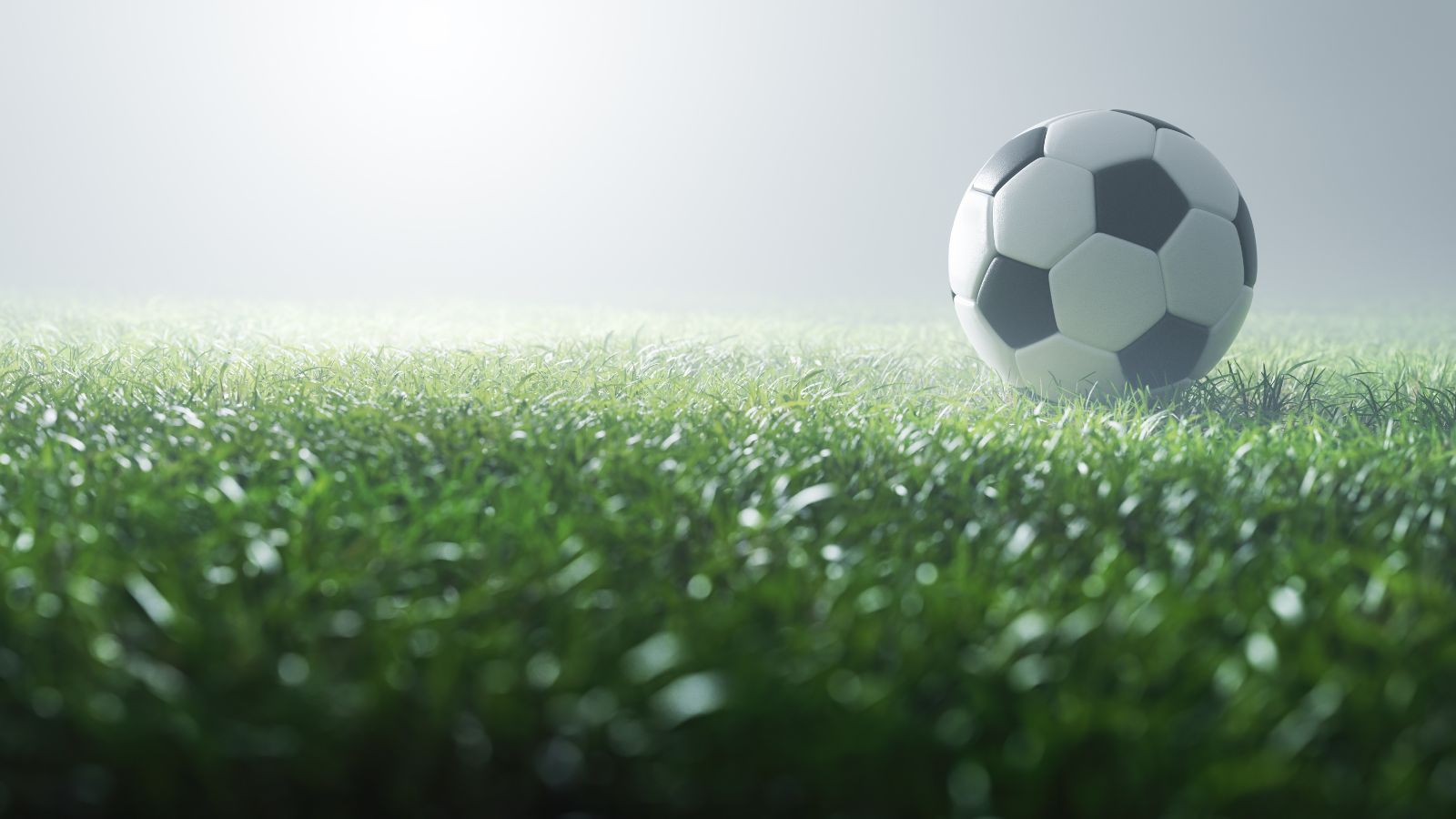
By examining these fundamental metrics, I gain a comprehensive understanding of each team’s strengths and weaknesses, allowing for more accurate predictions of match outcomes.
Data Collection and Processing
Sources of Data
When diving into predicting Champions League game outcomes using data science, I rely on a variety of reliable sources to gather the necessary data. These sources typically include official match reports, team statistics, player performance data, and historical match results. Additionally, I utilize reputable sports analytics platforms that offer detailed insights and metrics for a comprehensive analysis.
Cleaning and Preparing Data
Once I have collected the data from various sources, the next crucial step in the data science process is cleaning and preparing the data for analysis. This involves removing any inconsistencies, errors, or missing values from the dataset to ensure its accuracy and reliability. I also standardize the format of the data and perform feature engineering to create new variables that can enhance the predictive modeling process. By meticulously cleaning and preparing the data, I can optimize the accuracy of my predictions and extract valuable insights for forecasting Champions League game results.
Machine Learning Techniques in Sports Forecasting
Types of Algorithms Used
In sports forecasting, various machine learning algorithms play a crucial role in predicting game outcomes accurately.
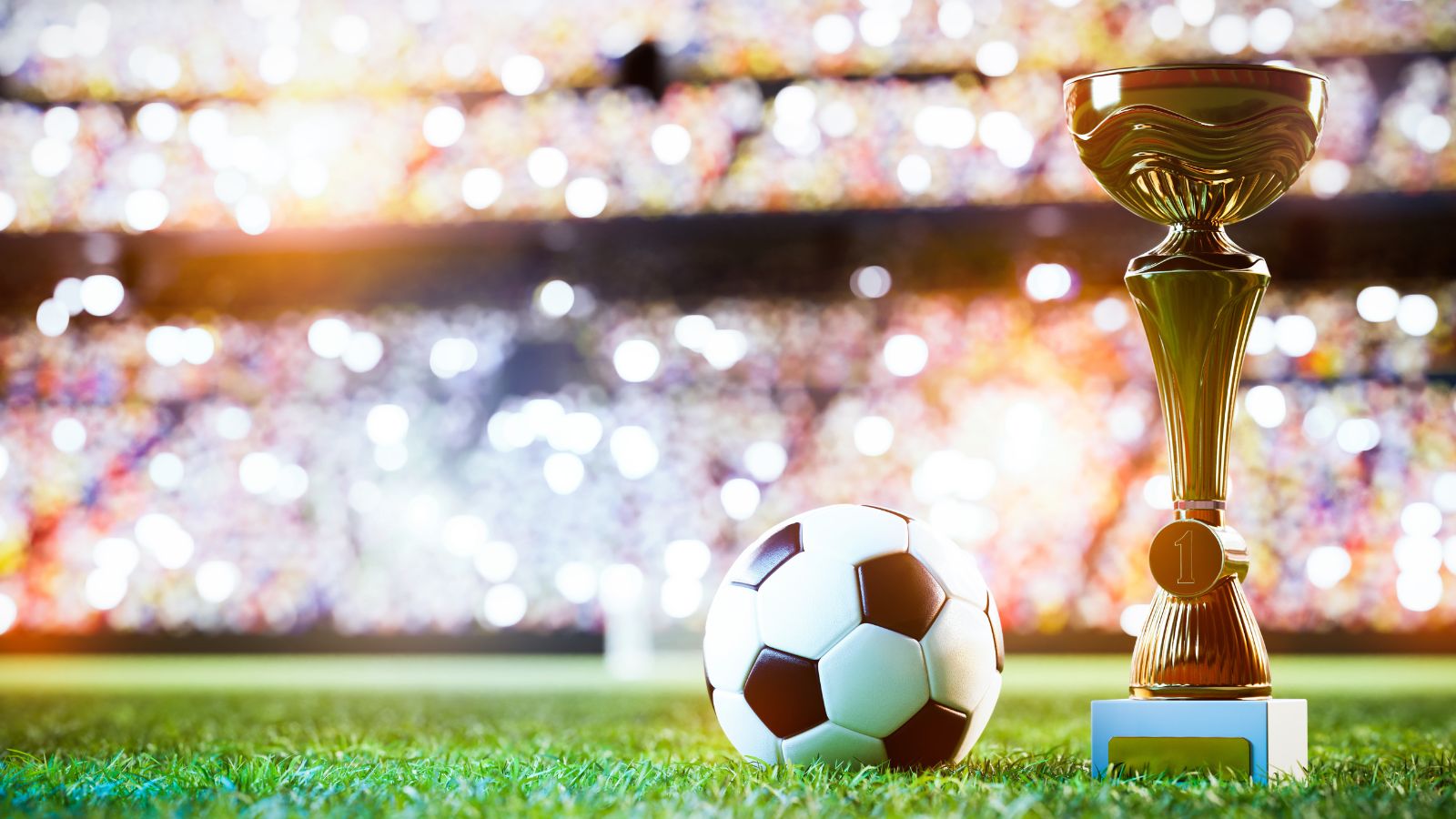
Algorithms such as Decision Trees, Random Forest, Support Vector Machines (SVM), and Neural Networks are commonly employed in analyzing historical data to forecast Champions League games. These algorithms process large amounts of data to identify patterns and trends, helping in generating reliable predictions.
Case Studies: Success Stories
Examining successful applications of machine learning in sports forecasting reveals remarkable outcomes. For instance, a study utilizing Random Forest algorithm for predicting football match results achieved an impressive accuracy rate of over 70%. Similarly, another case study implementing Neural Networks demonstrated significant success in forecasting player performance based on key performance indicators. These success stories underscore the effectiveness of machine learning techniques in sports analytics for generating precise forecasts.